Comparing Classification Methods for Mapping Invasive Floating Vegetation in the Sacramento Delta
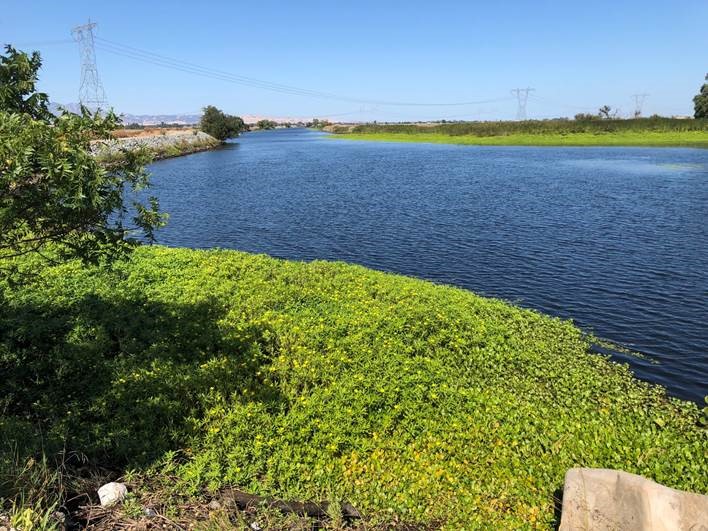
Abstract
Water hyacinth (Eichhornia crassipes) and water primrose (Ludwigia hexapetala) are aggressive invasive floating aquatic vegetation (FAV) species that cause severe economic and ecological impacts to the Sacramento-San Joaquin River Delta in California. These two invasive FAV occupy the same ecological niche within the delta ecosystem and form large floating vegetation mats made up of a mixture of both hyacinth and primrose. Remote sensing methods have proven to be successful in mapping and identifying invasive freshwater aquatic vegetation compared to traditional surveying methods. Using three different types of imagery (multispectral 2019 PlanetScope at 3.7m, multispectral 2018 WorldView-2 at 1.8m, and hyperspectral 2007 AVIRIS at 2.4m) four different remote sensing classification methods were performed to mapping invasive FAV - maximum likelihood (ML), support vector machine (SVM), object-based image analysis (OBIA), and spectral angle mapper (SAM) - resulting in six method/imagery combinations (Planet/ML, Planet/SVM, WorldView-2/ML, WorldView-2/OBIA, AVIRIS/ML, AVIRIS/SAM). The six classifications achieved producer’s accuracies from 83% to 91%. The AVIRIS/SAM combination resulted in the highest producer accuracy (91%) and the Planet/SVM combination resulted in the lowest producer accuracy (83%). This study revealed that, when mapping invasive FAV in a freshwater ecosystem, higher spatial resolution results in a higher classification accuracy. This study also revealed that when mapping FAV, hyperspectral imagery results in a higher classification accuracy than multispectral imagery.