Remote Sensing and Computer Vision Algorithms at Scale: Defense and Humanitarian Uses
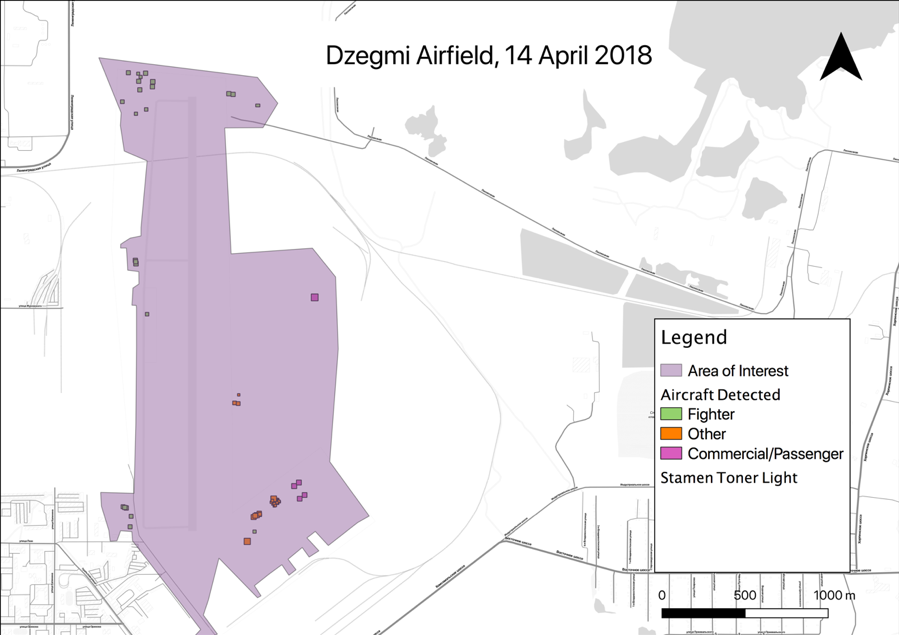
Abstract
The objective of this thesis is to understand how cloud computing and artificial
intelligence can be applied to vast amounts of remotely sensed data to better understand
macro-level trends for humanitarian and defense issues. Computer vision algorithms and
data were provided by Orbital Insight, Inc., a geospatial analytics company based in Palo
Alto, CA.
Specific projects were curated, data was acquired, and analysis was applied to
three use cases: “Patterns of life for The Battle of Marawi”, “Indications and Warnings
using multi-class aircraft detections”, and “Camp Fire land cover analysis”. The use cases
show how with imagery ingestion pipelines, cloud computing, and computer vision
algorithms, a massive quantity of data can be analyzed in a relatively short amount of
time. Without these workflows and new technologies, analysis of large amounts of data
would prove to be less efficient and resource heavy. The events show the benefits users
of spatial data would have to gain a better understanding of humanitarian and defense
issues. Algorithms used to derive insights from thousands of imagery scenes consisted of
a car detection algorithm, multi-class aircraft algorithm, and a land cover classification
algorithm. Additionally, the thesis briefly explores the use of geolocation data to
supplement computer-vision algorithm data. The thesis shows on a high level, through
examples, how users could use the technologies to analyze data more efficiently. This
analysis can be incorporated into high-level humanitarian or defense decisions. Future
work regarding this field should seek to evaluate the algorithm performance on a more
granular level. Researchers should also build different algorithms on open-source
imagery to allow for more users to benefit from the efficiency computer vision provides.